Modern time-lapse microscopy (TLM) has emerged as an effective and progressively refined instrument for investigating cellular biology and intercellular interactions, including applications that span from fundamental aspects of molecular and cell biology to medical practice. TLM empowers scientists to witness the dynamics and behaviors of not only living cell populations but also individual living cells within these populations. This article discusses the role of time-lapse microscopy in efficiently studying cellular dynamics.
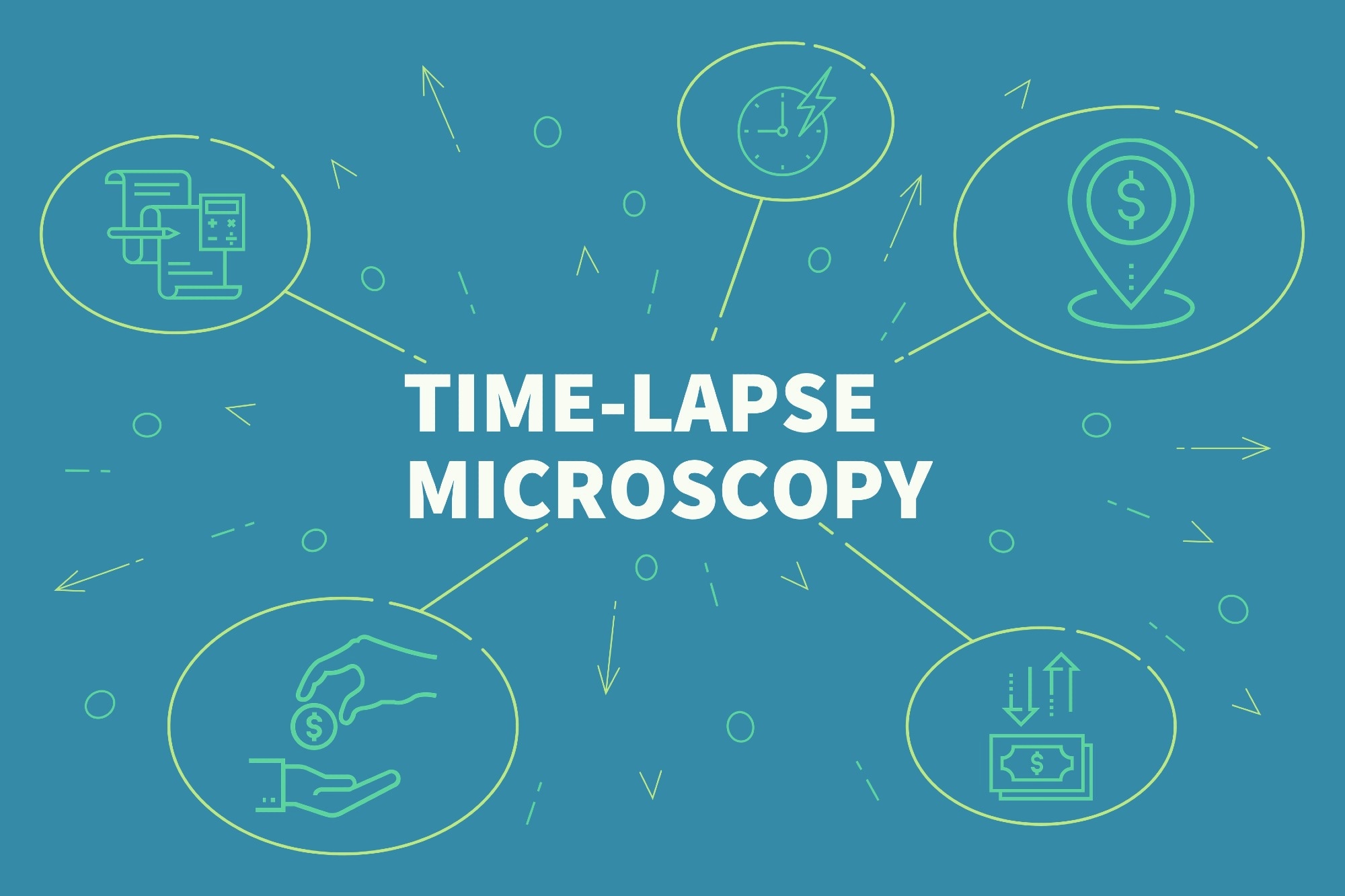
Image Credit: OpturaDesign/Shutterstock.com
A Brief Introduction to Time-Lapse Microscopy
Time-lapse microscopy (TLM) can be described as the process of capturing a sequence of microscopic images at regular intervals. In contrast to traditional microscopy, which yields static pictures of cellular structures, time-lapse microscopy reveals the dynamic state of cells and their operations.
The initial work related to live cell imaging and TLM techniques dates back to the early twentieth century. The advent of real-time imaging through TLM emerged as a pivotal breakthrough, overcoming a significant constraint of traditional microscopy necessitating thin, transparent samples that demanded tissue fixation and hindered the examination of living cells and biological processes over extended periods within the same sample.
Single-cell Segmentation Study by Time-Lapse Microscopy
Time-lapse microscopy is the only accurate technique capable of directly capturing the intricate dynamics and complexity of essential cellular processes at the individual-cell level, offering remarkable temporal precision. The effective utilization of single-cell time-lapse microscopy mandates the automated segmentation and tracking of numerous individual cells across multiple time instances.
The latest article in Cell Reports Methods states that the segmentation and tracking of individual cells continue to present challenges, notably in the analysis of time-lapse microscopy images, especially in the context of prevalent and non-invasive imaging modes like phase-contrast imaging. In recent times, deep learning (DL) has surpassed conventional rule-based image processing methodologies in tasks like object segmentation and object tracking.
DL-driven image processing approaches have garnered interest within the realms of cell biology and microscopy, particularly in the localization of individual molecules using super-resolution microscopy. Researchers have successfully devised a flexible and trainable DL model tailored for the segmentation of cell bodies and the tracking of cells within time-lapse microscopy images featuring mammalian cells at the single-cell level.
Named the "Deep Sea" model, this innovative approach facilitated researchers in tracking numerous cellular phenotypes and multiple cell division cycles across sequences of microscopy images. It enabled the creation of lineage tree structures for cells. Furthermore, the trained segmentation model expertly parametrized the precise boundaries of the target cells, assigning distinct colors to their pixels. This process aided in characterizing the shape and area of each cell within the input microscopy image.
Accurate Quantification of Phagocytic Events
Phagocytosis is a foundational cellular process wherein a cell engulfs substantial particles, including microbes and other cells. This process serves diverse functions, such as nourishment, tissue modification, and cellular defense. However, phagocytosis is characterized by its intensely dynamic nature, necessitating swift and extensive modifications.
As per the article published in the Journal of Cell Science, the existing techniques for quantifying phagocytosis rely massively on indirect or stationary assessments, such as target clearance or dye absorption. Consequently, these methods offer restricted insights into engulfment rates or target processing. Enhanced kinetic evaluations of phagocytosis hold the potential to furnish valuable fundamental insights across various domains.
The researchers have introduced a dynamic imaging approach involving live-cell, time-lapse high-content microscopy. This method is based on the identification and measurement of fluorescent dye "voids" within phagocytes. By leveraging this technique, it is possible to efficiently quantify optimal target cell densities and antibody concentrations essential for efficient antibody-dependent cellular phagocytosis.
Furthermore, the study compares void formation against dye uptake methodologies for phagocytosis detection while also scrutinizing the interrelation between target cell engulfment and phagolysosomal processing.
The findings revealed that this approach has the potential to accurately depict various stages of phagocytosis, as well as alterations in morphology that occur during phagocytosis, including both engulfment and target degradation. These outcomes develop a high-resolution avenue for quantifying phagocytosis, thereby opening up avenues to enhance comprehension of the intricate cellular and molecular coherence underlying this fundamental biological process.
Coupling TLM and Machine Learning (ML) for Cellular Dynamics Studies
Automated image acquisition systems have facilitated the execution of microscopic experiments, yielding extensive image datasets. However, a significant portion of microscopic techniques solely furnish intensity images and cannot discern the phase delay introduced by the cells being imaged.
To address this, an article published in the Journal of Biomedical Optics proposed a methodology for classifying cells during their transitions. This method involves time-lapse quantitative phase imaging (QPI) and has been demonstrated using the Namru Mus musculus mammary gland (NMuMG) cell line via the establishment of epithelial–mesenchymal transition (EMT) model system. Epithelial-mesenchymal transition (EMT) constitutes a pivotal process occurring during both developmental stages and pathological circumstances, particularly evident in fibrosis and wound healing.
In this experiment, cells were subjected to treatment with TGFβ (10 ng/ml). Following a 48-hour duration, cells under control conditions maintained their epithelial state, whereas cells treated with TGFβ underwent a transition to the mesenchymal state. The outcome data, in comparison to the conventional single-time-point approach, revealed that incorporating temporal information into the novel classification model for cell phenotypes during EMT led to an enhancement in performance of nearly 9% in terms of accuracy. This methodology has the potential to enhance the automated monitoring of live cell behavior.
Is TLM Effective for Studying Dynamics of Microbial Colonies?
In microbial communities, intricate interplays among microbial populations can significantly influence the collective characteristics of the community. This can result in cooperative behaviors and coexistence that benefit all parties involved.
For the investigation of interactions among bacterial biofilm populations on solid substrates, a methodology has been devised by researchers in the Microbiology Spectrum. This approach amalgamates features like microscopic precision, 3D observations, distinct labeling techniques, and uninterrupted imaging spanning up to 5 days. The efficacy of this approach, called BacLive, is accentuated by the utilization of top-tier long-working distance immersion optics.
The integration of high-quality long-working distance objective lenses and glass-bottomed petri dishes, in conjunction with bacteria labeled with fluorescence, facilitates the capture of substantial volumes of data. This data can be conveniently managed using the BacLive macro. This macro effectively streamlines the data collected during the image acquisition phase, thereby simplifying subsequent data analysis.
The integration of this approach with analysis tools like Imaris, BiofilmQ, or Comstat holds the potential to significantly contribute to the exploration of genetic processes within living bacteria. It also enables a more comprehensive understanding of the myriad interactions prevalent in the environment. Additionally, this strategy bears significance in applied sciences.
In summation, time-lapse microscopy has progressed massively within the realm of cell biology, aiding in the understanding of the dynamic intricacies within cells. As technological advancements continue to unfold, the prospects for the future of time-lapse microscopy are bright, offering the potential to delve even further into the complexities at the cellular scale.
Laser Speckle Contrast Imaging: Real-Time Visualization of Blood Flow and Tissue Perfusion
References and Further Reading
Zargari, A. et. al. (2023). DeepSea is an efficient deep-learning model for single-cell segmentation and tracking in time-lapse microscopy. Cell Reports Methods. Available at: https://doi.org/10.1016/j.crmeth.2023.100500
Chu, C. et. al. (2020). High-resolution quantification of discrete phagocytic events by live cell time-lapse high-content microscopy imaging. Journal of cell science, 133(5), jcs237883. Available at: https://doi.org/10.1242/jcs.237883
Strbkova, L. et. al. (2020). Automated interpretation of time-lapse quantitative phase image by machine learning to study cellular dynamics during epithelial–mesenchymal transition. Journal of Biomedical Optics, 25(8), 086502-086502. Available at: https://doi.org/10.1117/1.JBO.25.8.086502
Molina-Santiago, C. et. al. (2022). A Noninvasive Method for Time-Lapse Imaging of Microbial Interactions and Colony Dynamics. Microbiology Spectrum, 10(4), e00939-22. Available at: https://doi.org/10.1128/spectrum.00939-22
Disclaimer: The views expressed here are those of the author expressed in their private capacity and do not necessarily represent the views of AZoM.com Limited T/A AZoNetwork the owner and operator of this website. This disclaimer forms part of the Terms and conditions of use of this website.